Quantum AI The Next Big Leap
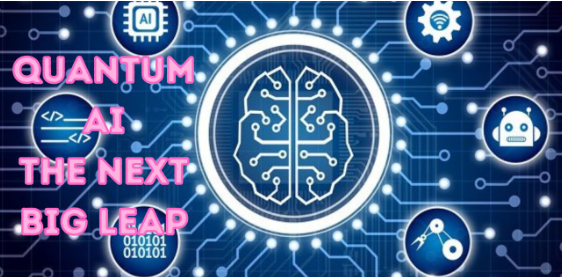
Introduction to Quantum AI
The fields of quantum computing and artificial intelligence (AI) were swiftly advancing main to the emergence of a brand new frontier Quantum AI. This fusion of quantum computing and AI holds the promise of revolutionizing how we technique complicated computational troubles, supplying unparalleled computational strength and capabilities.
At its centre, quantum AI harnesses the standards of quantum mechanics to beautify traditional AI algorithms and strategies. Quantum mechanics, the department of physics that describes the behaviour of debris on the smallest scales, introduces standards that include superposition and entanglement, which defy classical instinct but provide powerful tools for computation.
Traditionally, AI algorithms have trusted classical computers, which method records in binary bits, representing both a 0 or a 1. In assessment, quantum computers use quantum bits or qubits, which could exist in a couple of states simultaneously due to superposition. This inherent parallelism permits quantum computers to perform certain tasks a good deal quicker than classical computers.
Quantum AI leverages this quantum advantage to address complicated problems that can be beyond the attain of classical AI algorithms. By harnessing the precise houses of qubits, quantum AI algorithms can explore extensive solution spaces greater correctly, leading to breakthroughs in regions including optimization, machine gaining knowledge of, and data analysis.
However, the improvement of quantum AI continues to be in its early levels and lots of demanding situations lie in advance. Quantum computer systems are notoriously fragile, requiring specific management and isolation from external interference to maintain their quantum states. Moreover, building and scaling quantum hardware remains a vast engineering challenge, with modern quantum processors confined in size and coherence time.
Advantages and Challenges of Quantum AI
Quantum AI offers a plethora of advantages over classical AI approaches, but it also presents unique challenges that must be addressed for its widespread adoption.
Advantages:
1. Exponential Speedup:
One of the maximum benefits of quantum AI is its capability for exponential speedup over classical algorithms. Quantum algorithms can resolve certain issues lots faster than their classical counterparts through leveraging quantum parallelism and interference consequences. For responsibilities consisting of optimization, gadget learning, and cryptography, this speedup can unencumber new possibilities and enable breakthroughs in various fields.
2. Enhanced Exploration of Solution Spaces:
Quantum AI algorithms can explore massive answer spaces more effectively than classical algorithms. By leveraging quantum superposition and entanglement, these algorithms can simultaneously examine a couple of ability answers, main to quicker convergence and better basic overall performance. This functionality is specifically valuable for optimization issues, in which locating the highest quality solution amongst a huge set of opportunities is tough for classical algorithms.
3. Improved Data Processing:
Quantum AI can revolutionize facts processing by allowing faster and more green analysis of large datasets. Quantum algorithms for records clustering, type, and dimensionality reduction offer promising avenues for accelerating responsibilities which include pattern recognition, anomaly detection, and predictive modeling. By harnessing the electricity of quantum parallelism, those algorithms can extract meaningful insights from information greater quick, main to greater knowledgeable choice-making and progressed outcomes.
4. Quantum Machine Learning:
Quantum AI holds the promise of improving systems and getting to know strategies via leveraging quantum computing abilities. Quantum devices getting to know algorithms, such as quantum neural networks and quantum guide vector machines, offer new procedures for schooling and deploying AI models. These algorithms can make the most quantum entanglement and interference to perform duties which include feature selection, version education, and inference extra efficaciously, paving the way for greater effective and scalable machine studying systems.
Challenges:
1. Hardware Limitations:
One of the number one demanding situations facing quantum AI is the improvement of reliable and scalable quantum hardware. Quantum computer systems are notoriously touchy to environmental noise and decoherence, making it challenging to maintain the quantum states of qubits for prolonged periods. Current quantum processors are restricted in length, coherence time, and error charges, hindering their realistic software for actual international applications. Addressing these hardware boundaries requires advancements in qubit coherence, error correction, and fault-tolerance techniques.
2. Algorithm Design:
Designing quantum algorithms that outperform classical algorithms remains a complicated and challenging challenge. While quantum computing gives the capacity for exponential speedup, identifying troubles that can advantage of by this speedup and developing green quantum algorithms to remedy them is non-trivial. Quantum algorithms should be carefully crafted to leverage quantum parallelism and interference at the same time as mitigating the results of noise and decoherence. Moreover, translating classical algorithms into their quantum counterparts frequently calls for specialized information in both quantum mechanics and laptop technology.
3. Resource Requirements:
Quantum algorithms commonly require great computational resources, together with a large number of qubits and quantum gates, to obtain meaningful speedup over classical algorithms. As quantum hardware continues to adapt, scaling up the size and complexity of quantum algorithms remains a significant venture. Moreover, quantum algorithms may also require specialized ancilla qubits, mistake correction codes, and different sources to mitigate the effects of noise and mistakes, further increasing their resource necessities.
4. Algorithmic Robustness:
Quantum algorithms are inherently touchy to errors and imperfections in each hardware and software. Noise, decoherence, and different assets of mistakes can degrade the performance of quantum algorithms, restricting their practical utility for actual global applications. Developing sturdy quantum algorithms which might be resilient to noise and errors is vital for figuring out the full capability of quantum AI. This requires advancements in mistake correction techniques, fault tolerance mechanisms, and algorithmic robustness analysis.
Quantum AI Algorithms: Principles and Applications
Quantum AI algorithms constitute a fusion of quantum computing concepts with traditional synthetic intelligence techniques. These algorithms leverage the precise properties of quantum mechanics, consisting of superposition and entanglement, to carry out computations which are infeasible for classical computers. In this segment, we are able to discover the standards underlying quantum AI algorithms and their applications across diverse domains.
Principles of Quantum AI Algorithms:
Superposition:
Quantum AI algorithms exploit the precept of superposition, which lets in qubits to exist in a couple of states simultaneously. Unlike classical bits, which can handiest be in a nation of 0 or 1, qubits can represent a combination of both zero and 1 at the identical time. This allows quantum algorithms to discover multiple computational paths simultaneously, main to exponential speedup for certain issues.
Entanglement:
Quantum AI algorithms additionally harness the phenomenon of entanglement, which describes the robust correlation between the states of qubits. When qubits turn out to be entangled, the country of 1 qubit is intrinsically linked to the nation of every other, even when they’re bodily separated. This correlation lets in quantum algorithms to perform coordinated computations throughout more than one qubits, permitting complicated interactions and data processing.
Quantum Gates:
Similar to classical logic gates, quantum AI algorithms rely upon quantum gates to manipulate qubits and perform computational operations. Quantum gates follow unitary modifications to qubits, altering their states based on precise quantum algorithms. Examples of quantum gates encompass the Hadamard gate, which creates superposition, and the CNOT gate, which introduces entanglement among qubits. By making use of sequences of quantum gates, quantum algorithms can execute complex computations efficaciously.
Quantum Measurement:
Quantum AI algorithms finish with a quantum dimension, which collapses the superposition of qubits into classical states. This dimension yields probabilistic consequences based at the quantum states of the qubits, providing the effects of the computation. However, the final results of a quantum measurement is probabilistic, meaning that more than one runs of the set of rules may be required to attain dependable results.
Applications of Quantum AI Algorithms:
1. Optimization Problems:
Quantum AI algorithms excel at solving optimization issues across numerous domain names, along with logistics, finance, and production. By leveraging quantum parallelism, these algorithms can explore enormous solution spaces extra efficiently, permitting quicker and more accurate optimization. Applications include portfolio optimization, deliver chain management, and scheduling troubles, wherein finding the choicest answer is crucial for maximizing efficiency and minimizing expenses.
2. Machine Learning:
Quantum AI algorithms offer new tactics to device getting to know obligations, which include class, regression, and clustering. Quantum machine studying algorithms leverage quantum parallelism and entanglement to perform duties such as characteristic selection, version education, and inference greater effectively than classical algorithms. These algorithms keep promise for enhancing the performance of AI fashions and allowing new packages in regions including healthcare, finance, and herbal language processing.
3. Cryptography:
Quantum AI algorithms have implications for cryptography, specifically in the realm of quantum-secure cryptography. While quantum computers pose a hazard to classical cryptographic systems, quantum cryptography offers answers which can be proof against quantum assaults. Quantum algorithms for key distribution, encryption, and authentication leverage quantum concepts together with quantum key distribution and quantum-resistant cryptographic protocols to steady communications inside the quantum technology.
- Simulation and Modeling:
Quantum AI algorithms allow extra accurate and green simulation of quantum systems, including chemical reactions, materials houses, and quantum physics phenomena. Quantum simulation algorithms leverage quantum computing power to simulate the behavior of quantum structures, imparting insights into complex phenomena which might be difficult to look at the usage of classical strategies. Applications consist of drug discovery, materials layout, and quantum chemistry, where know-how quantum interactions is essential for advancing medical expertise and technological innovation.
Conclusion
In conclusion, the fusion of quantum computing and artificial intelligence represents a paradigm shift in computational technology and era. Quantum AI algorithms harness the precise homes of quantum mechanics to allow exponential speedup and better hassle-fixing talents. By leveraging quantum parallelism, superposition, and entanglement, those algorithms provide new methods to optimization, device gaining knowledge of, cryptography, and simulation.
While quantum AI holds wonderful promise for revolutionizing numerous domain names, it additionally affords full-size demanding situations. Overcoming hardware barriers, designing robust algorithms, and addressing resource requirements are essential steps in realizing the overall capacity of quantum AI. Despite these challenges, the potential programs of quantum AI are widespread and a ways-achieving, starting from optimizing supply chains and improving healthcare to advancing clinical studies and enhancing cybersecurity.